Antonios Parasyris, Sofia Darmaraki and Nikolaos A. Kampanis
Abstract:
In the context of the TexMed project we study the prediction of extreme warm seawater temperature events, named as Marine Heatwaves (MHWs), in the Mediterranean Sea. The basin is characterized as a climate change “hot spot” and MHWs pose additional threats on Mediterranean marine ecosystems that are already under stress due to anthropogenic activities. We therefore propose the implementation of machine learning (ML) techniques, as a new promising approach for the advance forecasting of these extreme climate events in the Mediterranean basin, where ML applications are still in their early stages. More specifically, a novel configuration of a U-Net Convolutional Neural Network (U-Net CNN) model is introduced for the short-term prediction (3 days and 7 days) of Sea Surface Temperature (SST) anomalies. To assess the model’s performance up to 7 days in advance, various factors are considered, including forecast horizons and input days. The proposed U-Net CNN model is shown to accurately predict the intensity and location of MHWs, featuring an average 8% improvement in Mean Absolute Error (MAE) compared to persistence climatological forecasts. Our results contribute further to the understanding of MHW occurrence in the basin. This innovative methodology has the potential to improve the timely prediction of MHWs, providing marine management authorities and stakeholders with valuable lead time for the implementation of effective mitigation strategies.
This project would be unfeasible to run on a standard personal computer, as it required the speedup of training Neural Networks in 4 GPUs in parallel at the GPU partition. Additionally, 230,000 CPU core hours have been spent in developing the required datasets that was used to derive the predictive models, utilizing both the high RAM of the server and the parallel computation of the CPU cores. Special thanks to MeluXina (https://docs.lxp.lu/) supercomputer for providing access to their CPU & GPU partitions.
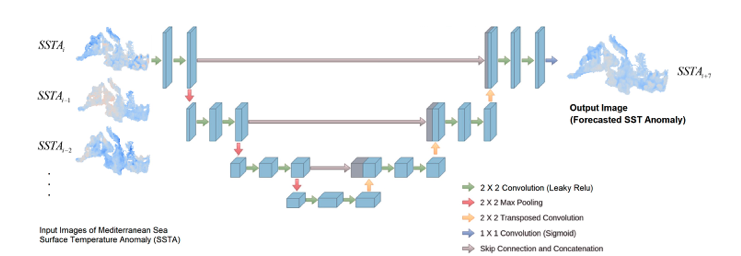
Figure 1: The proposed structure of our Unet that learns to predict Sea Surface Temperature anomalies in the Mediterranean Sea 1 week in advance.
Keywords: Marine heat waves; Mediterranean Sea; Machine learning; Convolutional Neural Network; extreme event forecasting; existence and severity; drivers